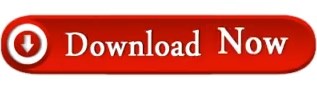

A good place to start is the Gajski-Kuhn Chart that outlines all the steps of chip design along three axes: the Behavioral level where architects defines what the chip is supposed to do, the Structural level where they determine how the chip is organized, and the Geometry level where engineers define how the chip is laid out. Before we take a look at these efforts, lets back up a little and look at the entire semiconductor design space. And Cadence Design Systems just announced an AI-based optimization platform similar to Synopsys DSO.ai.
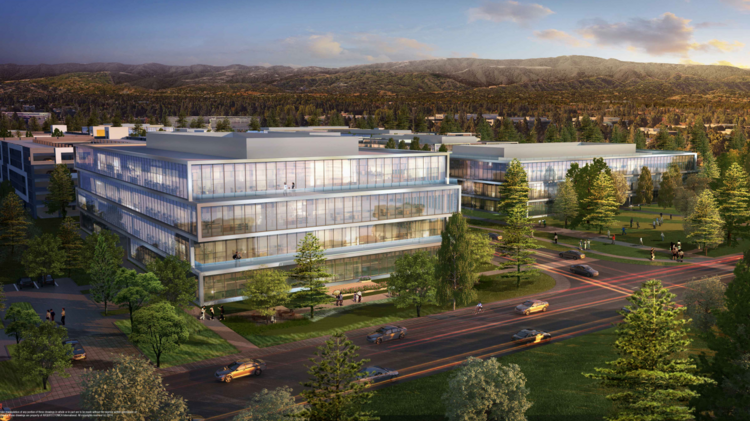
Recently Google published results of doing something similar, as has NVIDIA. Results are quite impressive, realizing 18% faster operating frequency at 21% lower power, while reducing engineering time from six months to as little as one. Since reinforcement learning can play Go better than the world champion, one could conceivably design a better chip if one is willing to spend the compute time to do it. The size of the problem/solution space DSO.ai addresses is staggering: there are something like 10 90,000 possible ways to place components on a chip. That compares to 10 360 possible moves in the game of Go which was mastered by Google AI in 2016.
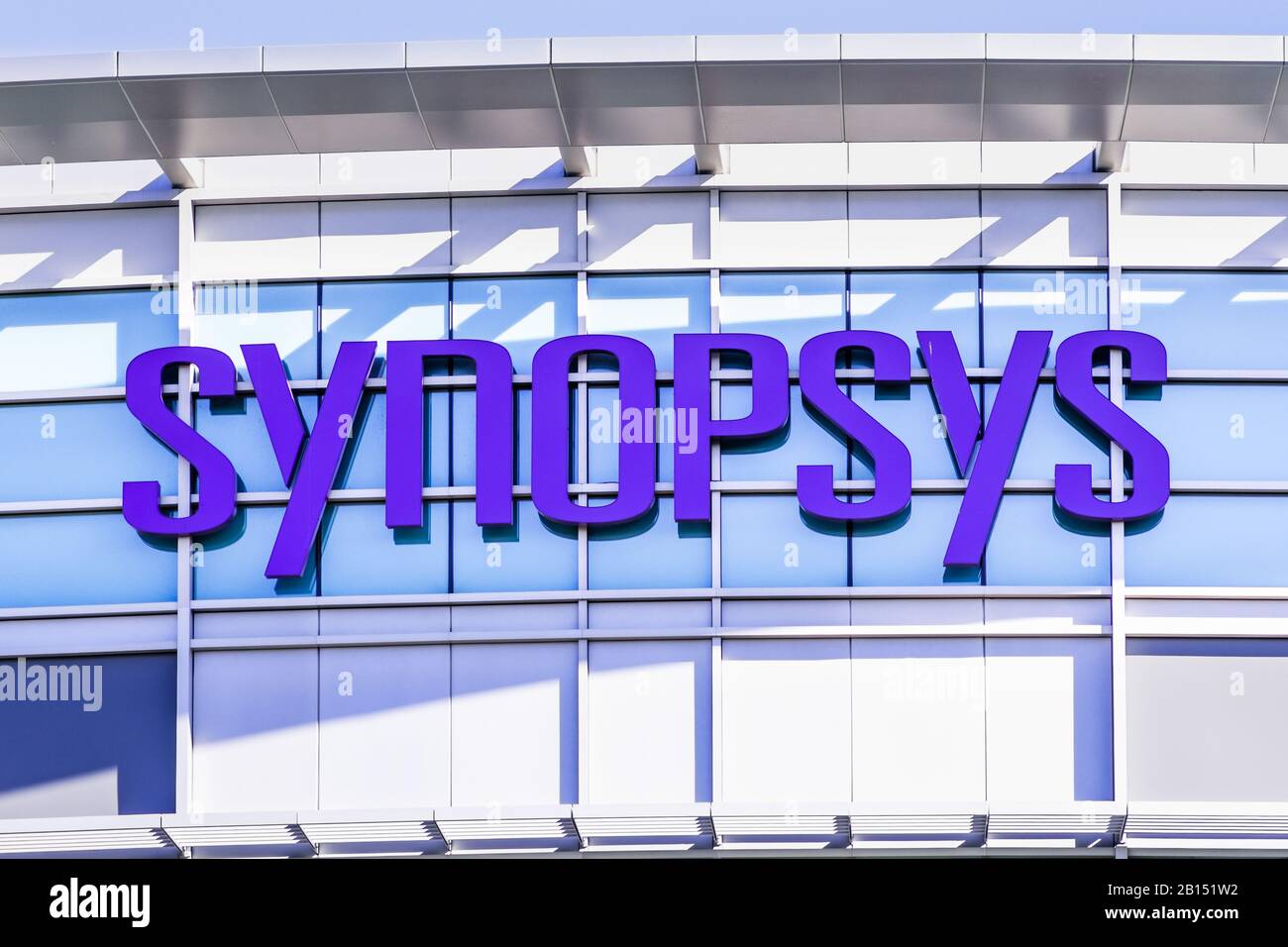
Using reinforcement learning, DSO.ai could evaluate billions of alternatives against design goals, and produce a design that was significantly better than that produced by talented engineers.
#Synopsys headquarters software#
This discussion began in earnest when the EDA leader Synopsys announced DSO.ai, Design Space Optimization AI, an software product that could more autonomously identify optimal ways to arrange silicon components (layouts) on a chip to reduce the area and reduce power consumption, even while increasing performance.
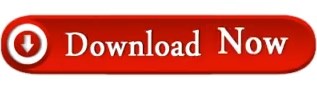